Chalearn LAP ConGD Database
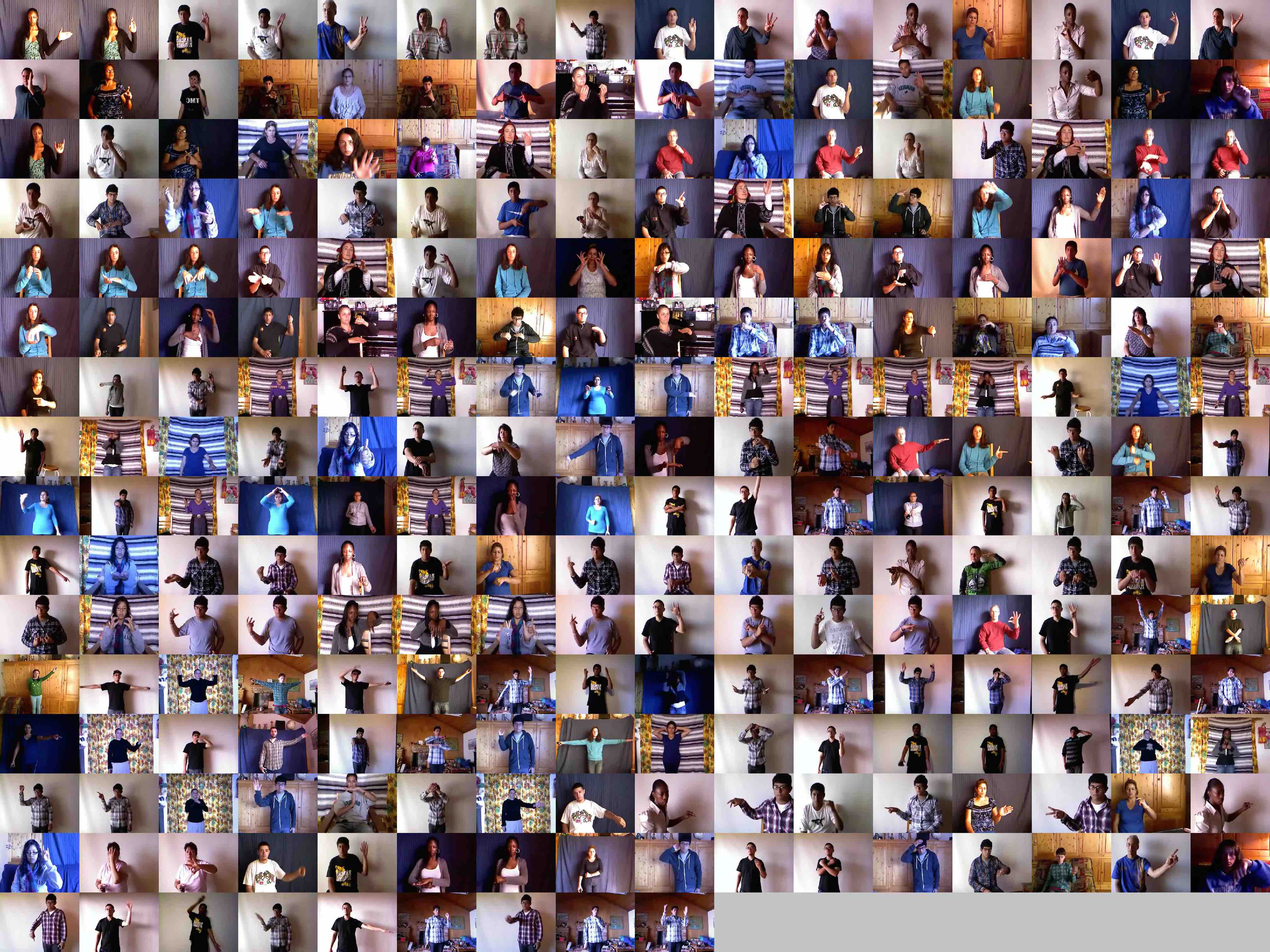
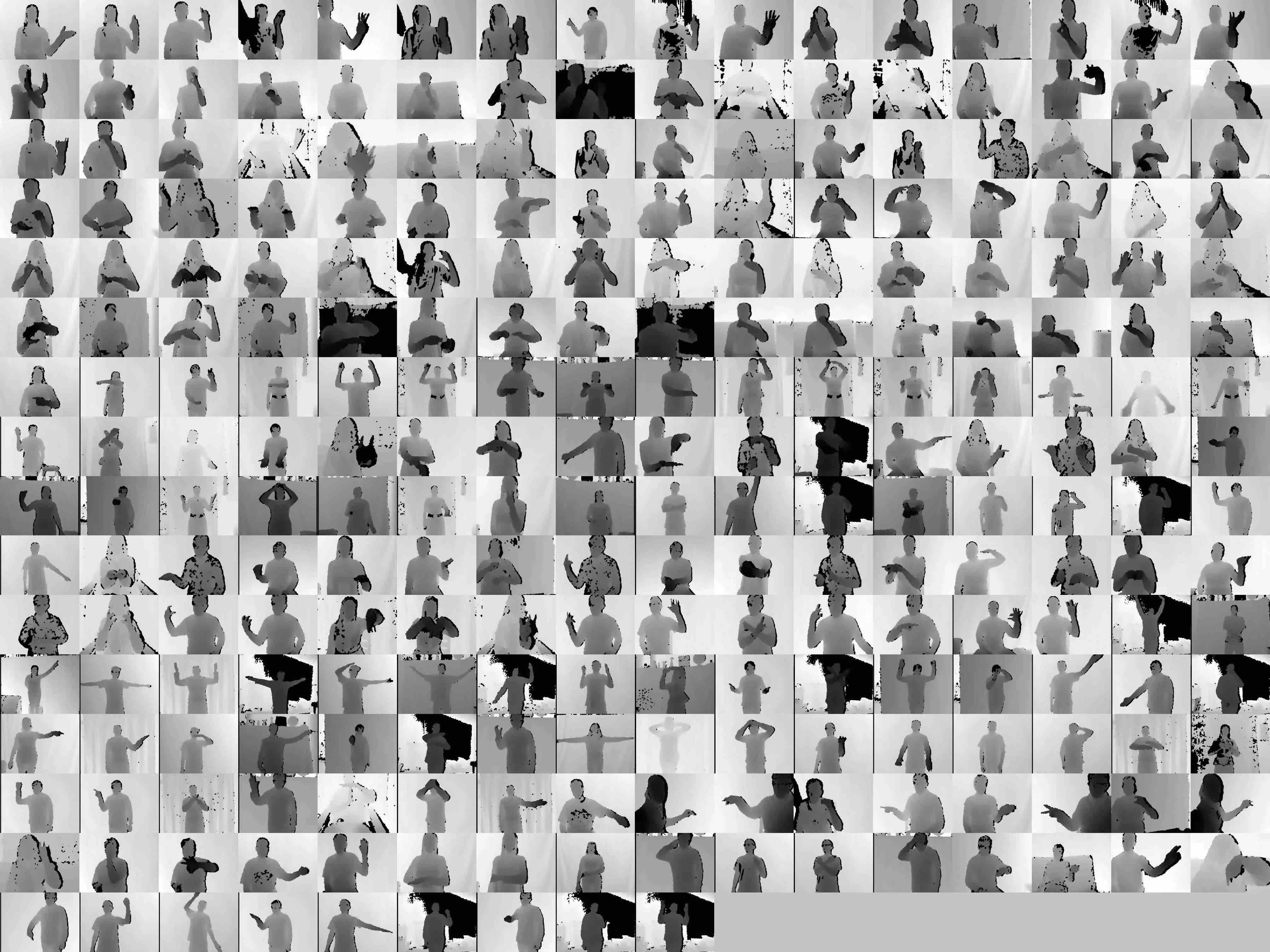
News: the test labels and related scripts are released: [download]
Introduction
Owing to the limited amount of training samples on the released gesture datasets, it is hard to apply them on real applications. Therefore, we have built a large-scale gesture dataset: Chalearn LAP RGB-D Continous Gesture Dataset (ConGD). The focus of the challenges is "large-scale" learning and "user independent", which means gestures per each class are more than 200 RGB and depth videos, and training samples from the same person do not appear in the validation and testing sets. The Chalearn LAP ConGD dataset is derived from the Chalearn Gesture Dataset (CGD) [1] that is used on "one-shot-learning". Because the CGD dataset has totally more than 54,000 gestures which are split into subtasks. To reuse the CGD dataset, we finally obtained 249 gesture labels and manually labeled temporal segmentation to obtain the start and end frames for each gesture in continuous videos from the CGD dataset.
Database Infomation and Format
This database includes 47933 RGB-D gestures in 22535 RGB-D gesture videos (about 4G). Each RGB-D video may represent one or more gestures, and there are 249 gestures labels performed by 21 different individuals.
The database has been divided to three sub-datasets for the convenience of using, and these three subsets are mutually exclusive.
Sets | # of Labels | # of Gestures | # of RGB Vidoes | # of Depth Vidoes | # of Performers | Label Provided | Temporal Segmentation Provided |
Training | 249 | 30442 | 14314 | 14314 | 17 | Yes | Yes |
Validation | 249 | 8889 | 4179 | 4179 | 2 | No | No |
Testing | 249 | 8602 | 4042 | 4042 | 2 | No | No |
Three .mat files were shipped with this database: train.mat, valid.mat and test.mat.
train.mat ==> Training Set . A structure array includes: train.video_name for RGB-D videos name,
train.label for the label information and
train.temproal_segment for the start and end points for each gesture in continuous videos.
valid.mat ==> Validation Set. A structure array includes: valid.video_name for RGB-D videos name,
valid.label for the label information (an empty cell array) and valid.temproal_segment
for the start and end points for each gesture in continuous videos (an empty cell array).
test.mat ==> Testing Set. A structure array includes: test.video_name for RGB-D videos name,
test.label for the label information (an empty cell array) and test.temproal_segment for the start
and end points for each gesture in continuous videos (an empty cell array).
Main Task:
1) Gesture spotting and recognition from continuous RGB and depth videos
2) Large-scale Learning
3) User Independent: the uses in training set will not disappear in testing and validation set.
Publication and Result
To use both datasets please cite:
Jun Wan, Yibing Zhao, Shuai Zhou, Isabelle Guyon, Sergio Escalera and Stan Z. Li, "ChaLearn Looking at People RGB-D Isolated and Continuous Datasets for Gesture Recognition", CVPR workshop, 2016. [PDF]
The above reference should be cited in all documents and papers that report experimental results based on the Chalearn LAP ConGD.
CONGD 2017:Rank | Team | r (valid set) | r (test set) |
---|---|---|---|
1 | ICT_NHCI [5] | 0.5163 | 0.6103 |
2 | AMRL [7] | 0.5957 | 0.5950 |
3 | PaFiFA [6] | 0.3646 | 0.3744 |
4 | Deepgesture [8] | 0.3190 | 0.3164 |
CONGD 2016:
Rank | Team | Mean Jaccard Index |
---|---|---|
1 | ICT_NHCI [3] | 0.2869 |
2 | TARDIS [4] | 0.2692 |
3 | AMRL [2] | 0.2655 |
- | baseline [1] | 0.1464 |
Ref:
[1] Jun Wan, Yibing Zhao, Shuai Zhou, Isabelle Guyon, Sergio Escalera and Stan Z. Li, "ChaLearn Looking at People RGB-D Isolated and Continuous Datasets for Gesture Recognition", CVPR workshop, 2016.
[2] Wang, Pichao, et al. "Large-scale continuous gesture recognition using convolutional neural networks." Pattern Recognition (ICPR), 2016 23rd International Conference on. IEEE, 2016.
[3] Chai, Xiujuan, et al. "Two streams recurrent neural networks for large-scale continuous gesture recognition." Pattern Recognition (ICPR), 2016 23rd International Conference on. IEEE, 2016.
[4] Camgoz, Necati Cihan, et al. "Using convolutional 3d neural networks for user-independent continuous gesture recognition." Pattern Recognition (ICPR), 2016 23rd International Conference on. IEEE, 2016.
[5] Liu, Zhipeng, et al. "Continuous Gesture Recognition With Hand-Oriented Spatiotemporal Feature." Proceedings of the IEEE Conference on Computer Vision and Pattern Recognition. 2017.
[6] Camgoz, Necati Cihan, Simon Hadfield, and Richard Bowden. "Particle Filter based Probabilistic Forced Alignment for Continuous Gesture Recognition." Proceedings of IEEE International Conference on Computer Vision Workshops (ICCVW) 2017. IEEE, 2017.
[7] Wang, Huogen, et al. "Large-Scale Multimodal Gesture Segmentation and Recognition Based on Convolutional Neural Networks." Proceedings of the IEEE Conference on Computer Vision and Pattern Recognition. 2017.
[8] Pigou, Lionel, Mieke Van Herreweghe, and Joni Dambre. "Gesture and Sign Language Recognition With Temporal Residual Networks." Proceedings of the IEEE Conference on Computer Vision and Pattern Recognition. 2017.
Evaluation Metric
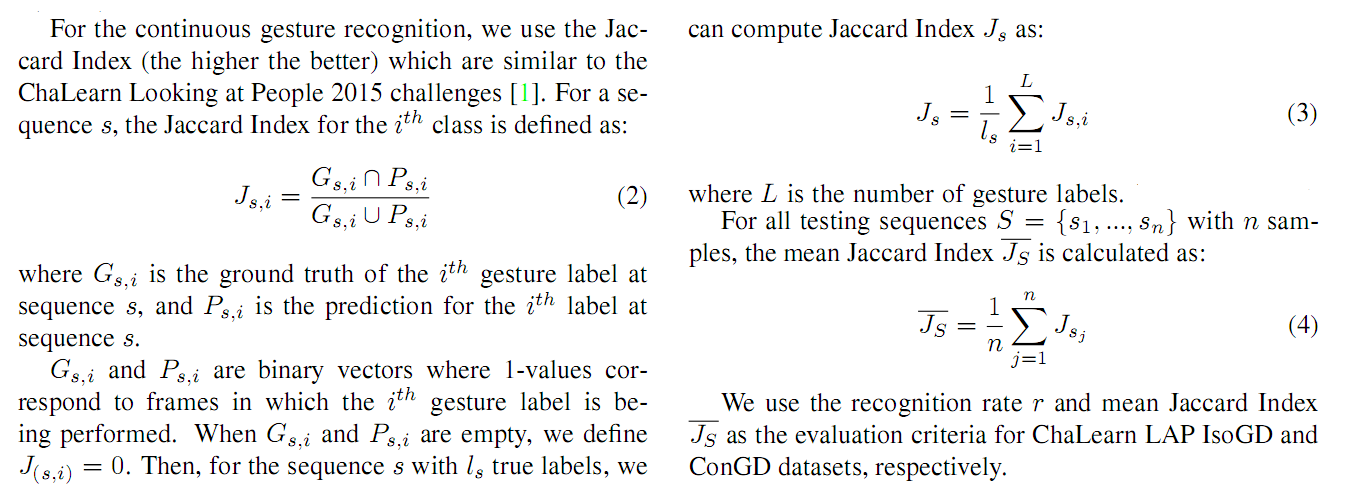
Download Instructions
To obtain the database, please follow the steps below:
- Download and print the document Agreement for using Chalearn LAP ConGD
- Sign the agreement
- Send the agreement to jun.wan@ia.ac.cn
- Check your email to find a login account and a password of our website after one day, if your application has been approved.
- Download the Chalearn LAP ConGD database from our website with the authorized account within 48 hours.
Copyright Note and Contacts
The database is released for research and educational purposes. We hold no liability for any undesirable consequences of using the database. All rights of the Chalearn LAP ConGD Database are reserved.
References
[1] Guyon, I., Athitsos, V., Jangyodsuk, P., Escalante, H. & Hamner, B. (2013). Results and analysis of the chalearn gesture challenge 2012.
Contact
Jun WanAssistant Professor
Room 1411, Intelligent Building
95 Zhongguancun Donglu,
Haidian District,
Beijing 100190, China.
Email:jun.wan at ia.ac.cn
joewan10 at gmail.com